AI and Machine Learning Set to Accelerate Natural Product Drug Discovery
-
Natural products are a rich source of chemical diversity and have yielded many important drugs, but discovering new natural product drugs has slowed. Advanced AI/ML methods are poised to accelerate natural product drug discovery by predicting biological activities, chemical structures, gene clusters, and more from the complex data.
-
Challenges remain in natural product drug discovery, including improving data quality and models, avoiding biases, ensuring reproducibility, and integrating the flood of genomic and chemical data. Community efforts for open data sharing, representation, and standards are critical.
-
Powerful deep learning methods allow for molecular property and bioactivity prediction, molecular graph representations, generative models for molecular design, and integration of chemical and biological data. But interpretability and evaluation on proper benchmarks are needed.
-
Integration of genomics, metabolomics, and other omics data enables linking biosynthetic gene clusters to natural products. Advanced AI/ML models leverage these connections for natural product discovery and predicting chemical structures.
-
Emerging community resources provide open access to mass spectrometry, NMR, metadata, chemical structures, bioactivities, and more to accelerate natural product research. But increased FAIRness, reproducibility, and integration are still required.
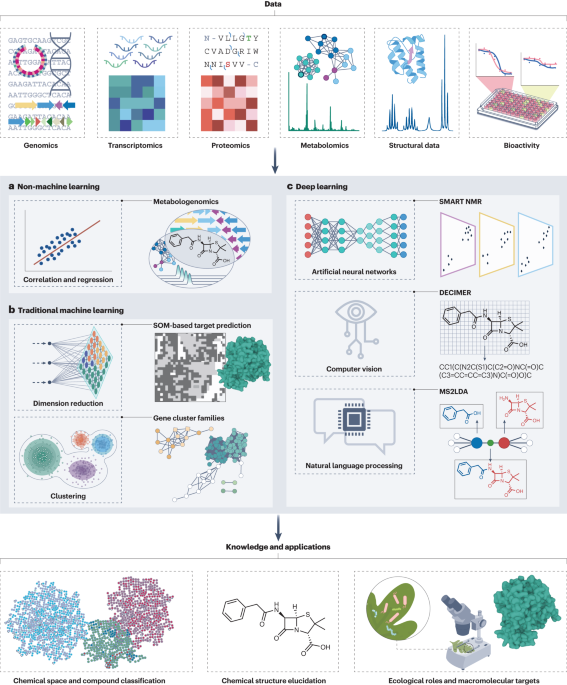